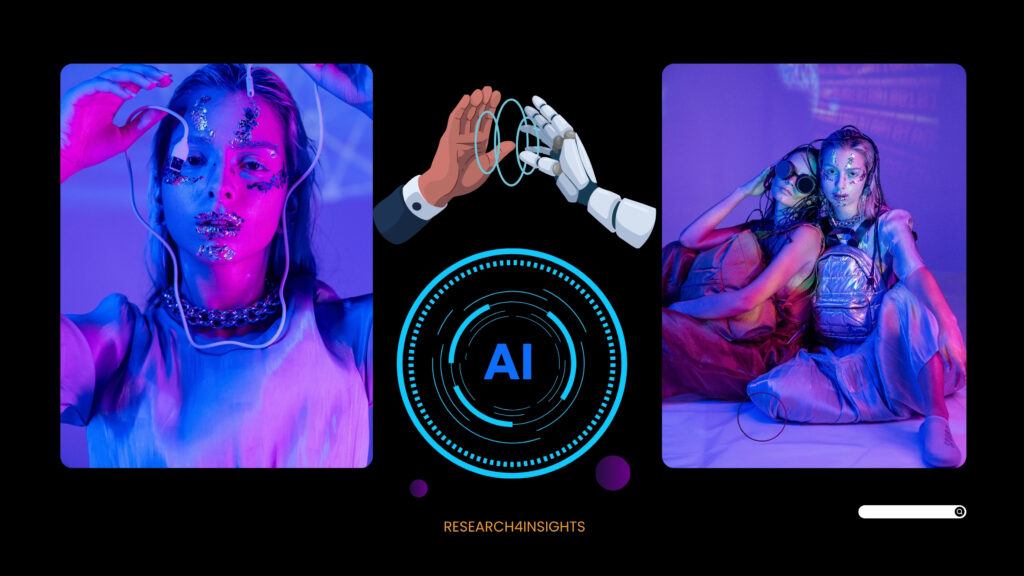
AI is revolutionizing many industries, and market research is no exception. However, very few organizations implement it to its best potential. More often, it is pitched as a substitution for human intelligence-substituting autonomous systems for complex workflows. Our experience has suggested a different approach-good AI systems do not intend to substitute expert human operators; they enhance and amplify them.
This corresponds to a far-reaching transformation in the relationship between research companies and technology partners. As more and more of the market research workflows get automated, the successful strategies have begun to move toward a different kind of collaboration-true partnership. In this model, rather than outsourcing, the guidance of human understanding helps drive development and improvement of AI systems, with AI taking care of the laborious repeat tasks once done by the researchers themselves.
It is a partnership between The Research4Insights and MaRel that exemplifies this philosophy. The first research firm to adopt MaRel’s AI-powered technology for survey data quality was The Research4Insights which developed a new model of human-in-the-loop development.
The approach in Study Into the AI Enhancing Data Quality in Market Research using MaRel and The Research4Insights partnership as a case study.
Further, we will discuss the particular problems this approach resolves, in addition to the technical and organizational reasons it does so effectively, alongside its broader implications for the future of market research.
Meeting Data Quality Issues in Market Research
Survey data quality has long been a large task facing market research. The Research4Insights employs an advanced methodology for data quality which includes digital fingerprinting, purposefully composed sampling, and treating survey data itself. This report will deal mainly with open-ended survey comments which in the past have been subjected to quality control through manual review of each comment by researchers, in order to review for potential problems. While effective, manual review was severely hampered by three inherent limitations: it was slow, could not scale to the fast-growing volume of responses, and was an acute cognitive load on researchers.
The AI Advantage
AI is remarkably good at automation of repetitive tasks and, moreover, in spotting subtle patterns undetectable by human experts. While the one way to deploy AI for better data quality does not directly imply a total takeover of the decision-making processes, it comes down to simplifying human knowledge…that is, the human element. To demonstrate this very philosophy, MaRel employs a full-fledged set of flags and indicators to help researchers direct their attention where it needs to be.
After assessing numerous rival products currently sold in the market, the Research4Insights has always found MaRel technology winning over them.
A Multi-Layered Approach to Quality Detection
MaRel approaches open-ended responses through a three-fold lens to search for a broader range of threats to data quality.
One of the issues faced in surveying is the low efforts invested by respondents in their submissions, such that responses yield little valuable information or insights. MaRel addresses this challenge with its content analysis that uses large language models to determine the meaningfulness of responses submitted to questions. Essentially, the project managers make more simplified accept/reject decisions, while MaRel hones in on granular flags such as “off-topic,” “gibberish,” and “low effort,” providing additional context as to why this particular response may be deemed low-quality.
Some coordinated fraud attempts at surveys include groups submitting similar responses or individuals making several submissions. MaRel’s syntactic analyses spot these patterns by clustering similar responses together. By clustering related responses together, researchers rapidly detect coordinated attempts and can review entire clusters in a single go.
Third, AI agents and bots corrupt survey data by injecting automated responses that introduce noise and bias. To counter this, MaRel employs behavioral biometrics to examine how participants interact with the survey page, analyzing things such as typing patterns, automated entries, and pasted content. These biometrics will catch such subtle patterns overlooked by human reviewers and flag automated responses that human review alone likely will miss. In this way, the joint use of AI pattern detection and human judgment can achieve a higher standard of data quality than either could ever reach alone.
Efficiency Through Precision
MaRel does not decide whether or not responses are good; rather, it is designed to flag responses that might warrant a closer review by a human analyst. Commonly, this type of review looks at 10-30% of responses, enabling testers to save up to 76% of the time otherwise spent checking open-ended responses, yet maintaining high standards.
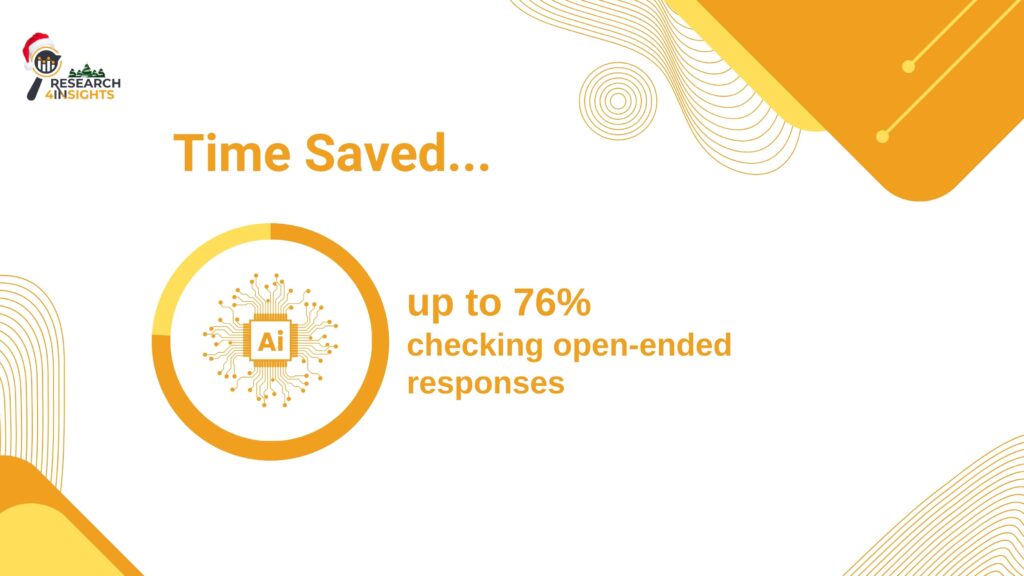
With time being saved by up to 76% in checking open-ended responses
This efficiency gain does not just save time but allows researchers more time to analyze the insights contained in high-quality responses themselves. They can make better judgments regarding edge cases when the routine quality checks are reduced. In effect, this creates a more efficient process, but it is also a more effective one, yielding much better quality data as research projects grow in scale and complexity.
Human-in-the-Loop AI: A Partnership Approach
Each of the component layers of MaRel’s system—content analysis, syntactic clustering, and behavioral biometrics—has been developed through a close partnership with The Research4Insights and tuned and refined afterward. This partnership is where the idea of Human in the Loop AI takes shape in two very important ways. The tools themselves are not built for full automation but rather for human use. As we will discuss below, the development of these tools arose from ongoing collaboration between MaRel and research experts at The Research4Insights.
Interactive Development
Combining the distinct strengths of the two companies: while The Research4Insights’s research team often finds minute quality issues that might go undetected by algorithms, MaRel performs a very deep technical analysis on those cases and draws lessons learned into their subsequent system updates. Thus, this virtuous cycle allows human insight to drive AI development, while better AI capabilities provide room for greater effectiveness in human oversight.
A recently brought to light instance shows how effective this approach can be. There were some that seemed to be suspicious responses to a question about the participant’s preferred temperature. Such responses not only contained unrealistic details that would hardly ever be found in authentic survey responses, such as very tidy estimates, conversion of temperature, and degree symbols, but had also successfully passed MaRel’s quality checks, although they became suspect to researchers at the Research4Insights.
MaRel’s analysis showed that these responses shared a distinctive pattern: they were entered with unnaturally consistent typing speeds and patterns unlikely to come from human participants. This insight led to the development of a new “unnatural typing” detector, which has now become a key component of MaRel’s data quality toolset.
Staying Ahead of Emerging Threats
This collaborative approach is so effective simply because issues of data quality are not static. New forms of fraud and quality issues surface almost daily, especially as AI tools are becoming easier to access. The human element in this human-in-the-loop process ensures that detection systems remain responsive to these evolving challenges. The human researcher detects new types of suspension behavior, while the AI system defaults this knowledge across millions of responses. Rather than operating independently, the technology provider and research firm work in tandem to build a symbiotic relationship that draws on the strengths offered by human insight and machine learning.
Conclusion: The Future of AI-Enhanced Market Research
Artificial intelligence is changing the business landscape across all industries. Of the many applications where AI can potentially assist the market research industry, none is more promising than addressing the issue of data quality, a long-standing challenge. However, as demonstrated through the partnership between MaRel and The Research4Insights, the most effective solutions don’t simply automate existing processes; they completely reimagine how researchers, AI, and technology firms can work together.
Under their ongoing partnership, researchers at The Research4Insights are now able to process more responses at a faster rate, giving expert consideration to those few cases that require human judgment. In the meantime, MaRel’s AI system has been continuously evolving in order to address new quality challenges, being constantly guided by the insights of experienced research professionals. This affords their end clients higher quality data at a faster pace and that allows The Research4Insights to fulfill the mounting demands for faster insight with improved quality.
Such a success illustrates a far larger opportunity in marketing research. As the capabilities of AI continue to evolve, the organizations that will prosper in the future will be those who can productively marry human intelligence with machine intelligence. The human-in-the-loop approach developed by MaRel and The Research4Insights gives a template for that future. In this model, technology providers and research firms work as true partners, continuously learning from each other for delivering better results for their clients.